- Autoencoder
- Generative Adversarial Network
- Diffusion Model
- Transformer Network
- Neural Radiance Fields(NeRFs)
- BERT model
- Large Language Model
Generative Artificial Intelligence
Generative AI
Generative Artificial Intelligence (AI) refers to AI systems or algorithms that have the ability to generate new content, such as images, music, text, or other forms of creative output. These systems are designed to learn patterns and structures from existing data and then generate new content that is similar to the training data.
Generative Adversarial Network : One popular approach to generative AI is using generative adversarial networks (GANs). The introduction of GANs in 2014 by Ian Goodfellow revolutionized generative AI. GANs consist of two neural networks: a generator network and a discriminator network. The generator network takes random noise as input and tries to generate realistic data, such as images. The discriminator network's task is to distinguish between real data and data generated by the generator network. Through an iterative process, the generator network learns to generate increasingly realistic data to fool the discriminator network.
Deep Learning Advances: The rapid advancements in deep learning, particularly in deep neural networks and convolutional neural networks (CNNs), have greatly contributed to the success of generative AI. Deep architectures enabled the modeling of complex patterns and structures in the data, resulting in more realistic and sophisticated generative models.
Application-Specific Models: Application-Specific Models: Over time, researchers have developed application-specific generative models tailored for specific tasks. For example, text generation has been addressed with models like recurrent neural networks (RNNs) and transformers, while image generation has seen the rise of models like GANs and VAEs.
Generative AI has a wide range of applications across various domains. Here are a few examples of how generative AI techniques are being applied:
- Natural Language Processing (NLP): NLP techniques are used to understand and process user input in natural language. This involves tasks such as text tokenization, part-of-speech tagging, entity recognition, sentiment analysis, and language understanding. NLP helps the AI system to interpret user queries and extract the relevant information needed to generate appropriate responses..
- Context Awareness: Responsive AI systems strive to understand and utilize contextual information to generate relevant responses. Context can include information about the user's previous interactions, preferences, location, or any other relevant factors. By considering context, the AI system can provide more personalized and tailored responses.
- Machine Learning and Deep Learning: Machine learning techniques, including deep learning, play a crucial role in building responsive AI systems. These techniques are used to train models on large datasets of conversations, allowing the AI system to learn patterns, understand user intent, and generate appropriate responses. Models such as recurrent neural networks (RNNs), transformers, and sequence-to-sequence models are commonly used in building conversational AI systems.
- Dialogue Management: Dialogue management involves handling the flow and structure of a conversation. It focuses on maintaining context, managing user interactions, and generating coherent responses. Techniques like state tracking and reinforcement learning can be employed to model and optimize the dialogue management process, ensuring smooth and meaningful conversations.
- Reinforcement Learning: Reinforcement learning can be employed to train responsive AI systems to interact with users effectively. By using reinforcement learning, the AI system can learn from user feedback and adapt its responses to maximize user satisfaction. Reinforcement learning enables the AI system to improve over time through an iterative learning process.
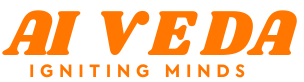